As per Gartner Report, by end of 2014, 70% of top 2000 global organizations would have implemented at least one Gamification application, however, by 2015, 80% of them will fail to meet business objectives due to poor design. 50% of organizations that have managed innovation processes will gamify those processes by 2015.
Therefore, there is a need for a Smart Gamification platform, as envisaged in the enclosed graphic, for sustained success.
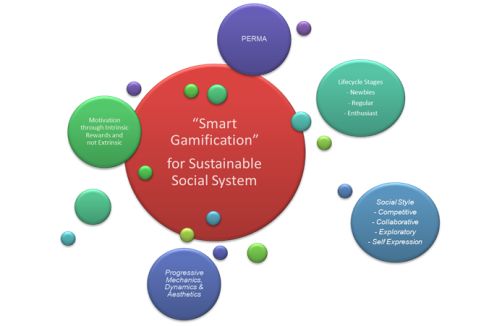
The key factors for adopting Gamification is to increase employee engagement.Engaged employees are happy that leads to better performance and that bundled with enhanced customer engagement leads to better customer experience and customer satisfaction. All this together increase employee and customer loyalty and better sales through positive WOM and referrals.
Having successfully implemented a Gamification BI platform for a large retail chain in US & Canada, and framework for a leading Sports Retailer in US, Happiest Minds has not only developed a Gamification Platform (combined with its own Campaign Management platform), but has also designed a Gamification Analytics platform with a logical data model, KPIs, etc.
Happiest Minds Analytics team is currentlyin the process of developing a Gamification BI & Analytics framework/solution that would help analyze the impact of any Gamification application on employees, customers and thereby help monitor, measure and optimize the designs, themes, etc. to make the Gamification platform most effective and sustainable.
A unique use case on application of Gamification proposed by Happiest Minds Analytics team aimed to improve quality of data coming from source systems into the data mart for a leading Middle East Infrastructure Conglomerate.
To explain the use case further – the customer had built an HR data mart to analyze some of the key metrics on regulatory compliance. Though a packaged BI solution had been implemented, some data quality rules had been embedded in the ETL code loading data into some of the subject areas of the data mart. The key issue remains that the number of rejected records being reloaded and remaining unclean is high and is affecting the success and utilization of the data mart that has been built.
The overall solution has 3 components:
- Developing a Data Quality Engine with Data Quality Monitoring Reports / Dashboards
- Improve the Data Entry Standard Operating Procedures and enable source system upgrade to ensure adherence to data quality and data governance
- Data Entry team to clean the current data (based on current criticality and priority) and thereafter to train the data entry stakeholder of the source system to ensure compliance to updated Standard Operating Procedures
The Gamification part of the solution includes building reject handling and historical reporting, and giving points to data entry operators who clean the data regularly and ensure compliance to established Standard Operating Procedures with reduced rejects and first time non rejects, first time complete corrections, etc.
A leaderboard would be built to provide real time or near-real time view of all leading departments and data entry operators and foster a healthy competitiveness to help in a larger cause. This would also then be rewarded in an intrinsic or extrinsic manner.
Bad data is detrimental to any business – whether its duplicates, default values, incorrect classification, typographical errors, inconsistent standards, inaccuracy or missing data, it would affect business decisions derived from reporting and analysis of enterprise data.
For example, an incorrect product description can lead to legal action, while incorrect pricing could lead to financial descent. In case of some industries like life science and healthcare industry or the infrastructure and auto industry, bad data can lead to life threatening consequences as well.
When inaccurate data is consumed by applications and users, errors are propagated across the enterprise thereby misleading higher level information, resulting in unnecessary risks when taking decisions. In short, garbage in should not be garbage out in the DWBI application!!
In our customer’s case, not only the Senior Management gets to take decisions based on accurate KPI information on resources, other functional departments including HR, Finance, various sub departments, project teams and IT team benefits in ways of enhanced resource management and optimization, reduced penalties, reduced cost of hiring, improved project planning, enhance IT service quality, etc.
Data Quality becomes a very critical aspect of post deployment maintenance of any DWBI Application. As a best practice, a data quality initiative should have already been a critical part of the DWBI Application development lifecycle, but quite often due to various constraints or over sightedness, ensuring data quality upfront is not a measurable functional requirement or success criteria of a DWBI application development effort, and is compromised as more of a cosmetic requirement that sometimes just remains limited to one-time profiling of the source data and some basic data cleansing rules bedded within the ETL process. Thus, post deployment of the DWBI solution, once the business users start seeing incorrect data coming in the reports and dashboards, Data Quality often becomes a very critical initiative and gets on a damage control modeto ensure that the usability and ROI of the DWBI system developed is realized.
Data Quality exercise should be started in parallel to the rest of the DWBI application design and development process ensuring that periodic data from the source system gets analyzed. This way, data cleansing, de-duplication, data standardization and even data governance could be established as part of the data transition process from the source to the final target data warehouse in the development cycle of the DWBI application.
Having said that, ensuring data quality is not a one-time process. Often in the DWBI application development cycle, data being analyzed for quality assurance is limited to the duration of the engagement and therefore test cases being designed for capturing anomalies, outliers and discrepancies in data only have a reasonable success probability in the AMS cycle. Periodic Data Quality check and reports should be therefore enabled in the AMS cycle.
Data quality should not be a symptomatic treatment of poor data quality being observed. It must be a more proactive measure based on exploratory data analysis and rectification of the root cause. Until the causal factors of poor data quality are analyzed and prevention measures are taken even to the extent of modifying the source application systems, the assurance on data quality cannot become predictable.
Data Quality is an ongoing mission journey more than a short term visionary drive. Gamification to improve data quality and sustainability therefore is not just necessary, it’s critical to the success of the initiative that involves people, processes and technology.
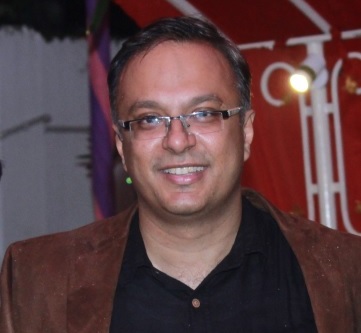
Sreejit has over 22 years of IT experience in Digital and Analytics leadership roles. Having setup the Digital Analytics Practice for Happiest Minds, Sreejit currently reports into Happiest Minds Executive Board (2 CEOs) for Strategic Accounts and Alliance Leadership. He is responsible for developing Strategies, Leading Alliances & Partnerships, Sales, Solutions Development & creating Non Linear Revenue Growth, Account Mining, and People Management. Sreejit is a B.Tech in Computers, a PMI certified Project Management Professional and has completed an Executive Management Program in Sales and Marketing from the Indian Institute of Management, Lucknow.